Dimensionality reduction (DR) is often used for exploring the evolution patterns of a collection of dynamic ego-networks. However, DR often lacks flexibility: as analysts' questions evolve during data exploration, the low-dimensional projection remains static, limiting the depth of exploration. To address the inflexibility of DR, we designed a data transformation pipeline which enables analysts to transform dynamic ego-networks into event sequences for steering MDS to create different scatterplots of dynamic ego-networks. Based on the data transformation pipeline, we developed Segue, a visual analytics system for exploring different evolution patterns of ego-networks.
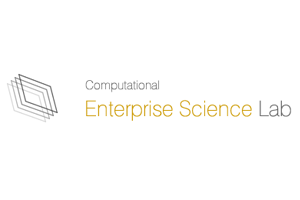
The Computational Enterprise Science Lab focuses on the design, analysis, and management of complex enterprise systems (e.g. organizations, supply chains, business ecosystems) using information visualization, modeling/simulation, and system science approaches.